Recent Posts
Archives
- February 2025
- January 2025
- December 2024
- November 2024
- September 2024
- June 2024
- May 2024
- April 2024
- March 2024
- February 2024
- January 2024
- December 2023
- November 2023
- October 2023
- September 2023
- June 2023
- May 2023
- April 2023
- March 2023
- February 2023
- January 2023
- December 2022
- November 2022
- October 2022
- September 2022
- August 2022
- May 2022
- April 2022
- March 2022
- February 2022
- January 2022
- December 2021
- November 2021
- October 2021
- September 2021
- August 2021
- July 2021
- May 2021
- April 2021
- March 2021
- February 2021
- January 2021
- December 2020
- November 2020
- October 2020
- September 2020
- August 2020
Categories
For CSE Students: Microsoft Azure AI Fundamentals Training on Oct 17-18
October 5, 2021 / Leave a comment
As part of its collaboration with Qatar University, Microsoft is inviting our CSE students to attend a virtual training on Artificial Intelligence (AI) Fundamentals where you can discover the solutions that are possible to create with AI and the Azure services that can help you build those solutions. The training will be held on Oct 17 & 18 (9:30 AM – 12:30 PM). Below is the training agenda and the MS Teams link to join it.
Agenda
|
Microsoft Teams meeting
Join on your computer or mobile app
Click here to join the meeting
Or call in (audio only)
+44 20 7660 8187,,822662347# United Kingdom, London
Phone Conference ID: 822 662 347#
PDRA7 Award to CSE Faculty and Postdoc
September 24, 2021 / Leave a comment
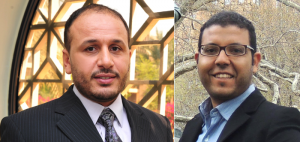
Prof. Khaled Shaban, as a Primary Research Mentor, and Dr. Alaa Abdellatif, as a Postdoctoral Fellow, are awarded two years funding for their proposal in the seventh cycle of Postdoctoral Research Award (PDRA) from Qatar National Research Fund (QNRF). The project is titled “AI-driven Secured Demand Response in Next Generation Smart Grid Using Blockchain and 5G Networks”. It is one of two PDRA proposals awarded by QNRF to Qatar University in this cycle. More details on the project can be found here.
Qatar Seeds for the Future 2021
September 19, 2021 / Leave a comment
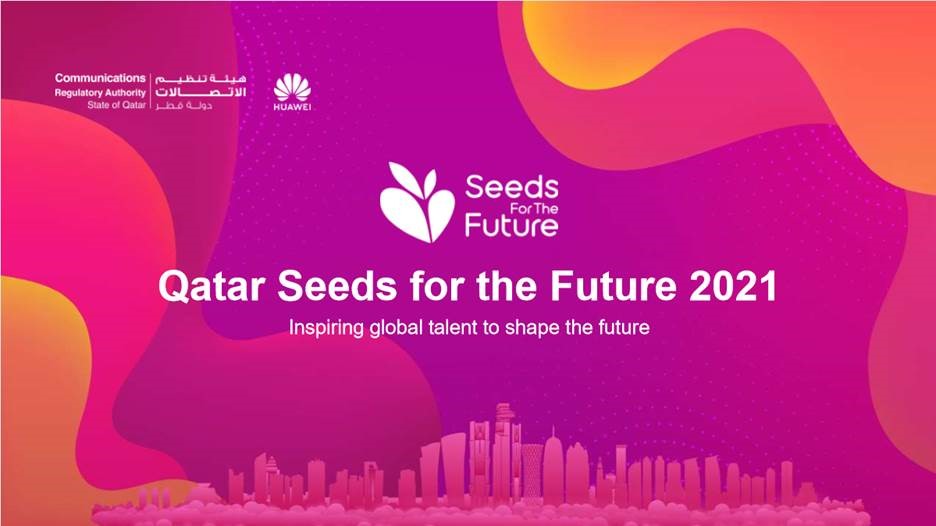
Under the endorsement of Qatar Communication Regulatory Authority, Huawei Technologies organized its 4th edition of “Seeds for the Future” program over 8-day online training from 23 to 30 August 2021 for Qatar top students to obtain valuable cutting-edge ICT knowledge developed by Huawei’s Headquarters. The program offered a rich experience involving technology courses including 5G, cloud computing, AI, IoT, leadership course, and participation in “Tech4Good” group project.
This year 31 students from QU, HBKU, and CCQ have participated in the program and so proudly QU students represented by CSE department have achieved the highest number of distributed awards. Following is the complete list of our CSE-QU student winners for each award category:
Best Tech4Good Project: Abhigyan Kishor, Fatemeh Ahmadizdeh, Ziad Abdelazim Sabry Abdelazim, Ifran Ahmed Rafi, Kulsum Kader, Noof Mohammed Ali Hassan Qassmi, Sofia Basha
Daily Best Student: Moslem Al Qawasmi, MahnoorAkhund, ZainehAbughazzah
Best Team Leader: Kulsum Kader
Best Performance: Amira Radwan Menisy Abdalla, Fatma Elnahas
Social Media: Moslem Al Qawasmi
Top 5 Students: Islam Hamdi, Sofia Basha
Big congratulations for all our wining students and wishing them the bright future in their study and beyond!
CSE Faculty Participates in Facebook “Ethics in AI Research Initiative for the Asia Pacific” Roundtable
September 10, 2021 / Leave a comment
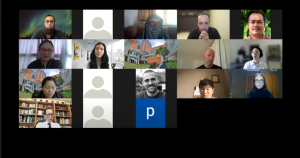
Dr. Junaid Qadir, Professor of Computer Engineering at our department participated in the “Facebook Ethics in AI Research Initiative for the Asia Pacific” Roundtable on 10th September, 2021, and presented an update on the “Culturally-informed pro-social AI regulation and persuasion framework” project. This project is funded by Facebook Research and focuses on studying the ethical issues related to Artificial Intelligence (AI) from an Islamic Perspective and explores culturally-sensitive human-centered AI for the Muslim World. The roundtable was attended by top AI experts and discussants included ethicists, technologists, researchers, and policy makers.
Dr. Junaid is currently teaching the course CMPE483 “Introduction to Robotics” at QU. The course provides a detailed overview of the robotic ecosystem and discusses basic concepts related to sensing, actuation, and robotic intelligence. The course also explores the ethics of using AI and intelligent robots and its socio-technical implications.
We are Hiring!!!
September 2, 2021 / Leave a comment
We are looking for:
Assistant/Associate/Full professor
The Computer Science and Engineering Department is seeking high-caliber candidate for faculty position at the levels of assistant, associate, and full professor. The applicant should possess a Ph.D. degree in Computer Engineering, or a related field from a reputable university and should demonstrate a strong record in teaching, research, and services. While applicants in all areas of computing will be considered, the following areas are of particular interest to the department: Robotics, cybersecurity, AI for hardware design, embedded systems, biomedical engineering, quantum computing. The positions are available beginning January 2024.
More information is available in the attached file: Here
Lecturer
The applicant should hold a Ph.D. degree in Computer Science or an equivalent field from a reputable university. Moreover, the candidate must have a proven track record in teaching courses relevant to Mobile Application Development, Web Development, and Security (e.g., applied cryptography). We are particularly interested in individuals who are highly motivated and eager to collaborate with our dynamic team. The position is available starting from January 2024.
More information is available in the attached file: Here
How To Apply:
- New applicant: The application needs to set up an account here and complete the personal profile and apply to the selected position.
- Returning applicant: The applicant should log in to his/her account in here and update first his/her information and then apply.
- The IDs of the different positions are as follows:
Position ID
Assistant/Associate/Full professor | IRC21041 |
Lecturer | IRC25450 |
Note: The department currently is not seeking any applicants for any other positions.
The position is available beginning January 2024.
New Patent Granted for CSE Faculty in Collaboration with KINDI Center
August 11, 2021 / Leave a comment
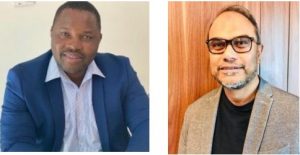
Dr. Khaled Khan and Dr. Noora Fetais from the Department of Computer Science and Engineering (CSE) have been awarded a patent titled “Methods and Systems for Monitoring Network Security” in collaboration with KINDI Center for Computing Research in the College of Engineering at Qatar University.
The Qatar National Research Funds has supported this research under National Priority Research Program (NPRP8-531-1-111). Dr. Khaled and Dr Noora acted as the Lead PI and PI of the project respectively. Dr. Armstrong Nhlabatsi from KINDI also participated in the research project. They collaborated with the researchers from the University of Canterbury (New Zealand) and the University of Queensland (Australia).
Modern networks are becoming more and more dynamic, such as frequent changes compared to the traditional static networks (e.g., hosts addition and removal, vulnerability change, applications and services update, and attack surface change). In a dynamic network, the configuration of at least one of the hosts or edges changes over time. The dynamic network is a network selected from a group of configured components including cloud computing, a software-defined networking arrangement, or an Internet of Things network. As network components change over time, the security posture of the network also changes, as vulnerabilities associated with the network components shift accordingly. Hence, it is of paramount importance to be able to assess the security of dynamic networks in order to understand and further enhance the security.
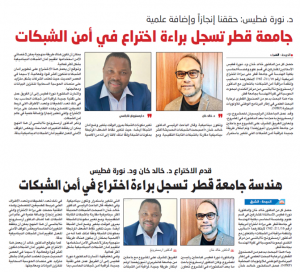
This patented approach is a new technique for monitoring the security of a computing network, which includes a plurality of hosts and a plurality of edges which link connected hosts. The method comprises capturing and storing network state information in response to at least one of the time-driven trigger, an event-driven trigger or a user-driven trigger. The method further comprises storing security-related data which is indicative of the change in the security of the network during the time window for a user to monitor the change in the security of the network. Detecting a change in the security of the network comprises at least one of the following events: the addition of a new host to the network; the removal of a host from the network; the addition of a new edge to the network; the removal of an edge from the network; the addition of a vulnerability to a host in the network; or the removal of a vulnerability from a host in the network. The method calculates at least one security metric for the network and a weight value for at least one of the components in the network. The approach is capable of capturing such critical changes and reflecting the modified security posture in order to precisely assess the security of dynamic networks.
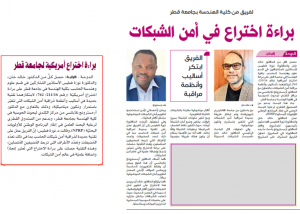