Five of our faculty members (Dr. Noora Fetais, Dr. Abdelaziz Bouras, Dr. Mahmoud Barhamgi, Dr.Khaled Shaban, and Dr. Elias Yacoub) and their research teams have just been been awarded QU internal grants 2024 (Cycle 7) in the different categories. Congratulations!
Here are more details about the winning projects:
Award Category: Collaborative grants (CG)
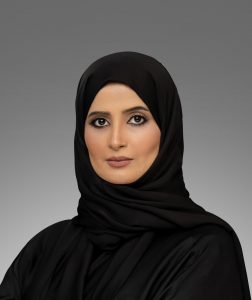
Project title: Practical Adversarial Machine Learning for Network Intrusion Detection Systems
Project team: LPI: Dr. Noora Fetais (QU), PIs: Dr. Khaled Khan (QU), Dr. Armstrong Nhlabatsi (QU), Consultant: Dr. Dan Dongseong Kim (University of Queensland)
Project abstract:
An adversarial example exploits an imperceptible attribute of the input to cause a deep-learning (DL) algorithm to misclassify. Attackers intentionally design these adversarial examples to confuse the model so that it makes a mistake. Some researchers hypothesize that adversarial examples are caused by highly abstract representations, which make the decision function extremely discontinuous. In contrast, others claim that adversarial examples occur due to the locally linear nature of neural networks. As a result of adversarial examples, researchers are developing countermeasures to defend against adversarial attacks against DL-based applications, especially in security-critical domains such as Network Intrusion Detection Systems (NIDS). However, the security of NIDS under adversarial attacks has not been well explored. The aim of this research is to enhance the understanding of practical adversarial attacks on NIDS. Here, a “practical” adversarial attack means the output of the attack is a set of replayable network packets, and adversarial attacks specifically refer to evasion attacks that slightly modify the input to bypass detection. To achieve these goals, this research proposal seeks to:
- Develop an adversarial attack generator tailored for NIDS and present a comprehensive evaluation framework to determine the severity of the attack on the NIDS.
- Propose a defense mechanism to defend against adversarial attacks and formulate another evaluation framework to examine the strength of the defense.
The proposed project is expected to bridge previously identified limitations in adversarial NIDS attacks/defenses. To enable automation and deployment of future attacks/defenses, software toolbox/frameworks will be designed and developed. More importantly, the resulting framework, methods, techniques, and tools of this project are expected to be readily applicable in real world settings.
Award Category: Collaboration Co-Fund (IRCC) Grants
Project title: MESledger: A decentralized intelligent control of production systems based on AI and Blockchain technologies.
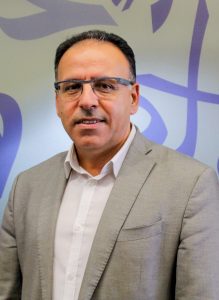
Project team: LPI: Dr. Abdelaziz Bouras (QU), PIs: Dr. Khaled Benfriha and Améziane Aoussat (ENSAM Institute of technology, Paris-France). Dr. Abdelhak Belhi (JBJADS, Qatar). Dr. Mahmoud Barhamgi and Dr. Loay Ismael Sabry (QU)
Project Abstract: The project focuses on the development of a new system for the intelligent control of digitalized production systems. Indeed, the old production control systems, based essentially on automats (Programable Logical Controllers), are not adapted to the new manufacturing processes, said digitalized. Such new system will be confronted with a mass of data generated by the IoT layer and the various IT systems required for operation.
It becomes important to think about a new “manufacturing execution system” able to analyze the data, to order dynamically the operations and to take the adequate decisions in front of malfunctions. Moreover, such system will be able to optimize production operations according to priorities, such as cost, energy or production time. To do this, it would be necessary to review the design of a manufacturing operation, known as standard and parametric. Thus, the system should be intelligent enough to configure the values of the parameters according to the priorities.
The industrial stakes are high. Companies will not be able to reach the expected performances without the intelligent exploitation of the generated data. From a scientific point of view, the challenge is to develop intelligent and scalable algorithms that can drive a digital production system with a high level of autonomy.
Project title: Building Transparent, Fair and Privacy-preserving Smart City Applications
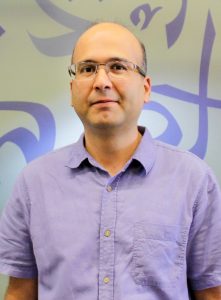
Project team: LPI: Dr. Mahmoud Barhamgi (QU), PIs: Dr. Saeed Salem, Dr. Qutaibah m. Malluhi, Dr. Noora Fetais (QU), Dr. Daniela Grigory (Paris-Dauhine University, France), Dr. David Camacho (Madrid University, Spain)
Project abstract: In Qatar, as well as in all advanced countries, Intelligent Cyber-Physical Systems (ICPSs) are increasingly becoming an integral part of people’s life. Their applications are exploited today to optimize many aspects of our daily lives including in healthcare (e.g., remote patient monitoring networks), smart food supply chains, smart road infrastructures (e.g. for efficient real-time regulation of traffic), and smart grids (e.g., for greener energy consumption). All of these applications are collectively called smart city applications.
They collect huge amounts of data about us, process and exploit them to make important decisions that affect our lives both positively or negatively. As these smart systems and applications are expected to grow and stay with us, it is important to ensure they are designed and operationalized in a way to respect the fundamental rights of their users.
In this project, we focus on how three fundamental rights of users including Transparency, Fairness, and Privacy can be ensured in ICPSs. Specifically, we propose models, mechanisms and software tools allowing the users of such systems to monitor how and why a decision affecting them was taken by the system, based on what data as well as how fairly they have been treated by the system compared to their peers or other groups of users. The provided justifications are computed by our solution while respecting the privacy of all users or data subjects impacted by the system. We intend to apply and validate our solution in two specific smart city applications including smart patient monitoring and traffic optimization.
Project title: DeIN: A Drone-based Inspection System for Outdoor Insulators in Qatar.
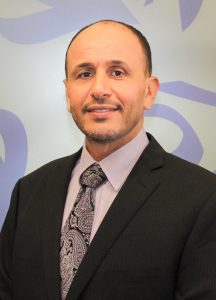
Project team: LPI: Dr. Khaled Shaban (QU), PIs: Dr. Saeed Salem (QU), Dr. Ayman El-Hag (University of Waterloo)
Project abstract: This research proposal aims to enhance the effectiveness of inspection systems for outdoor, high voltage insulators utilized in the electric power transmission networks of Qatar, the Gulf region, and beyond. The proposed research is significant due to the criticality of outdoor insulators in supporting and insulating overhead power lines (OHPL) and the impact of their poor electrical performance on the resilience of the entire transmission network. Insulators are subjected to harsh environmental factors such as hot and humid weather, heavy dust and sand depositions, and salt buildup, leading to accelerated aging and increased maintenance costs. Insulators are responsible for over 70% of power line outages and require continuous monitoring to prevent premature failures. While traditional inspection methods are expensive, time-intensive, and laborious, aerial-based robots, such as drones, equipped with sensors and advanced algorithms offer a promising solution. However, the data obtained from drones is susceptible to noise and uncertainties, affecting their accuracy and limiting their inspection capabilities. The proposed solution, DeIN, aims to develop a novel drone-based inspection system that combines vision and radiation-based sensors with state-of-the-art representation learning, fusion, and deep learning (DL) algorithms. DeIN offers advantages such as reduced inspection time and cost, increased personnel safety, enhanced precision, repeatability, and improved access to OHPL, eliminating user subjectivity. Moreover, the use of radiation-based sensors provides enhanced inspection capabilities for detecting surface cracks and internal voids, complementing the vision-based sensors. The proposed research contributes to the development of a reliable and efficient inspection system for high voltage insulators in Qatar, the Gulf region, and beyond, ensuring the reliability and security of power transmission infrastructure.
Award Category: CD-IRCC Sustainable Development Goals (SDGs)/Local Projects)
Project title: Wearable Bracelet and Machine Learning for Remote Diagnosis and Pandemic Infection Detection.
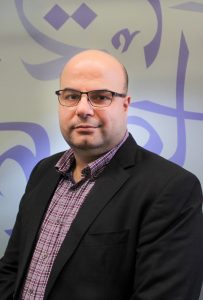
Project team: LPI: Prof. Elias Yaacoub (QU), PIs: Dr. Ahmed Badawy and Dr. Khalid Abualsaud (QU)
Project abstract: More than three years into the coronavirus disease 2019 (COVID-19) pandemic, it can be noted that the measures put in place for societies to manage the spread of this disease could have been better. For example, contact tracing mobile applications used to curb the spread of COVID-19 need additional enhancements to allow health care professionals to better understand the proliferation of the disease and to lessen the burden on hospitals and medical centers. In this project, we present an intelligent solution to remotely self-monitor COVID-19 symptoms to help rapidly identify and detect suspected positives. The proposed intelligent solution is based on using a near-field communications (NFC) wristband that collects body temperature, heart rate and SpO2 levels. It is connected to a dedicated mobile application to intelligently draw conclusions from the data (COVID-19 symptoms) it collects. Moreover, the application is trained to analyze cough sounds and detect the probability of infection. Current results show more than 90% of detection accuracy. The work in the project aims to deliver a prototype at TRL 5 or 6, where the bracelet with embedded sensors will be fully functional and the readings are sent to the mobile application, where they can be jointly analyzed with coughing sounds to further enhance performance. The proposed system can be adapted to future pandemics based on respiratory symptoms.