A team from our CSE department has received the Best Paper Award in the 10th International Conference on Bio-inspired Systems and Signal Processing (BIOSIGNAL2023) conference with a paper titled “Wearable Data Generation Using Time-Series Generative Adversarial Networks for Hydration Monitoring”. The team includes Farida Sabry, Dr. Wadha Labda (LP), Eng. Tamer Eltaras, Ms. Fatima Hamza, and Dr. Qutaibah Malluhi. in collaboration with Dr. Khawla Elzoubi from Community College of Qatar.
BIOSIGNAL 2023 brought together researchers and practitioners from multiple areas of expertise working at the intersection of engineering, mathematics, statistics, computer science, data science, biology and medicine, who develop and apply algorithmic tools, models and techniques to solve challenging problems in biology and medicine. A diversity of signal types can be found in this area, including video, audio, electrophysiological signals, medical imaging, and other biological sources of information. The analysis and use of the diverse types of data seem across these applications often requires cross-disciplinary expertise and collaborative efforts and this conference aims to be a high quality forum to celebrate many of these ongoing interactions and research efforts.
Paper Abstract: Collection of biosignals data from wearable devices for machine learning tasks can sometimes be expensive and time-consuming and may violate privacy policies and regulations. Successful and accurate generation of these signals can help in many wearable devices applications as well as overcoming the privacy concerns accompanied with healthcare data. Generative adversarial networks (GANs) have been used successfully in generating images in data-limited situations. Using GANs for generating other types of data has been actively researched in the last few years. In this paper, we investigate the possibility of using a time-series GAN (TimeGAN) to generate wearable devices data for a hydration monitoring task to predict the last drinking time of a user. Challenges encountered in the case of biosignals generation and state-of-the-art methods for evaluation of the generated signals are discussed. Results have shown the applicability of using TimeGAN for this task based on quantitative and visual qualitative metrics. Limitations on the quality of the generated signals were highlighted with suggesting ways for improvement.
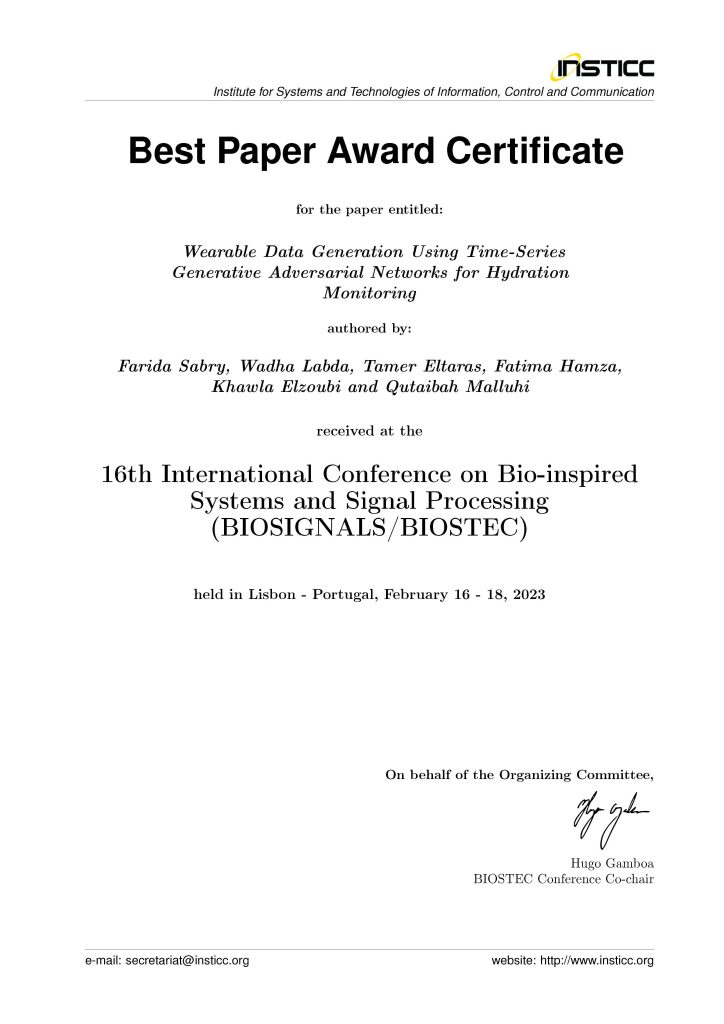