Our CSE department has just been awarded three Academic Research Grants (ARG) from the Qatar National Research Fund (QNRF) in its inaugural round. The three awarded projects are led by Prof. Amr Mohamed, Prof. Somaya Almaadeed, and Dr. Ahmed Badawy.
Here are more details about the awarded projects:
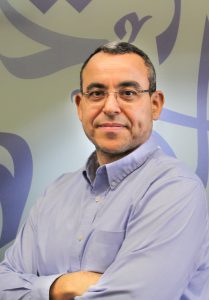
The PervasiveAeroAgents platform’s primary objectives are designed to address important challenges facing disaster scenarios, including 1) establishing the system architecture and describing coordinated multi-drone features such as sensing specifications, wireless charging, intelligent detection algorithms, and autonomous navigation. 2) Developing AI-based computer vision techniques using machine learning, to detect and identify objects and individuals among the debris, while using Reinforcement learning (RL) and online learning (OL) techniques for autonomous navigation, speeding up search and rescue operations in stochastic and highly changing environments. 3) Developing new security protocols suitable for dynamic ad hoc group communications amongst the drones to guarantee integrity and confidentiality. Finally, 4) build a comprehensive proof-of-concept using digital twin technology to demonstrate system features and insure the efficacy of the proposed sensing and AI-based techniques for group ad hoc communication.
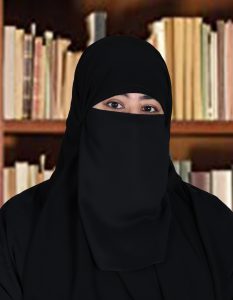
Project abstract: Cancer, which has been identified as a significant public health issue in Qatar and worldwide, can be diagnosed early and accurately with the help of biomedical imaging. It is true that there has been a significant increase in cases of breast, thyroid, colon, prostate, lung, and stomach cancer over the past five years [1]. For instance, Qatar has one of the highest rates of female breast cancer incidence and mortality when compared to the other Middle Eastern regions. In Qatar, the latest Qatar National Cancer Registry (QNCR) report of 2020 revealed that breast cancer had the highest incidence among all types of cancers. It accounted for 37% of all cancer cases, with 218 new cases reported. Colorectal cancer ranked second among female cancers, comprising 10.7% of cases with 62 reported instances. Thyroid cancer held the third position, representing 7.2% of female cancer cases with 42 reported cases [2]. Due to a variety of factors, including lifestyle choices, environmental effects, and other factors, there are an increasing number of cases of breast cancer in Qatar and the surrounding nations. Imaging and biomedical imaging techniques, such as histology image [3], and/or positron emission mammography (PEM) for breast cancer screening [4], are frequently used by caregivers to accurately detect the spread of cancer in the human body. To locate, segment, and categorize malignant tumors, these biomedical imaging approaches rely on image processing and Artificial Intelligence (AI) [4]. Both AI and computational imaging and analytics for cancer detection layer of these imaging approaches are not sufficient to provide accurate diagnose of cancer and doctors do not understand the science behind the result. Therefore, we need a much smarter way to explain and hence link the results to the clinical data. Together with medical doctors in Qatar and UAE we aim to develop new tools and techniques for multimodal breast tumor classification based on integrative data analysis from imaging and clinical data including histopathological and OMICs. We aim to develop explainable AI tools to outline how the AI produced a certain result. AI can be used as a support system that scans image and process corresponding clinical data by extracting the areas, features, or data with a high probability of cancer to simplify a doctor’s job and provide additional hints for medical care and competence. Furthermore, this proposal aims to decipher the molecular pathogenesis of breast cancer using artificial intelligence through integration of histopathological images and OMICs data from different breast cancer subtypes. Multimodal data fusion of morphology, gene expression, and DNA mutations using IHC and OMICs technology has yet to be explored in depth. Implementing this approach using AI and Deep Learning (DL) can lead to a more accurate diagnosis of the disease and timely treatment. This will improve their overall survival and decrease the economic burden of breast cancer.
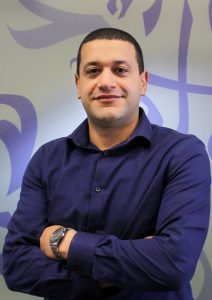
Project abstract: The Open Radio Access Network (O-RAN) is on track to completely transform the telecommunications ecosystem in the coming decade. O-RAN specifications are expected to drive 50% of RAN-based revenues by 2028 for public networks and by 2027 for enterprise and industrial cellular segments and will exceed traditional RAN by 2030. This research proposal aims to investigate and develop a reliable O-RAN framework for time-critical and high-resource-demanding healthcare applications.